In today’s digital economy, data-driven innovation is reshaping industries, and product management is at the forefront of this transformation. Leveraging data allows product managers to make informed decisions, craft better customer experiences, and drive product evolution in ways previously unimaginable. In this blog, we’ll explore several key ways to implement data-driven innovation in product management to optimize outcomes, boost agility, and stay ahead of the competition.
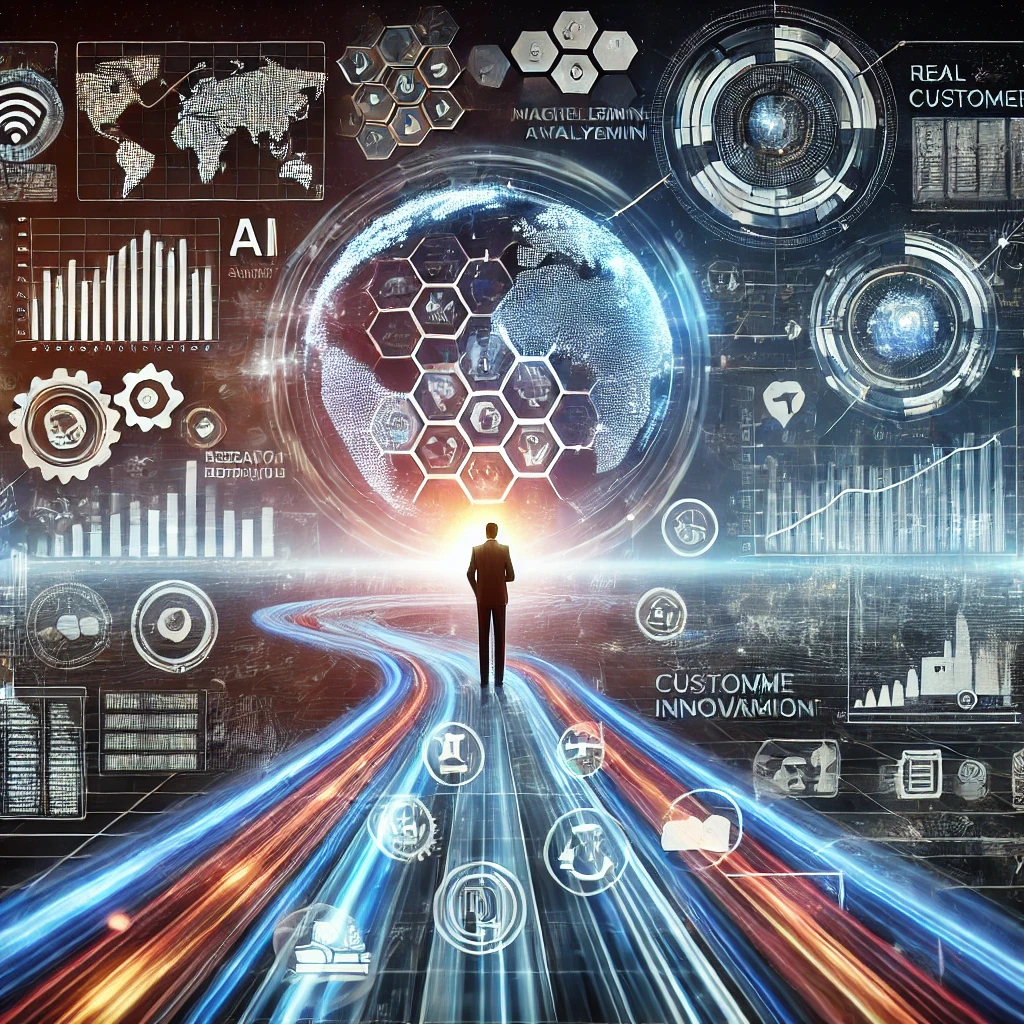
1. Implementing Real-Time Data Analytics
The adoption of real-time data analytics allows product managers to make timely decisions. Real-time data reveals how users interact with products, and how these interactions change in various scenarios. Tracking real-time usage metrics, customer engagement levels, or product performance KPIs empowers teams to respond quickly to potential issues, refine the product roadmap, and capitalize on emerging trends.
Eg: E-commerce platforms use real-time data to monitor buying behavior, enabling them to suggest products based on the user’s current interaction history, boosting sales and improving the overall shopping experience.
2. Customer Feedback Loops for Continuous Improvement
Incorporating customer feedback into the product development cycle is essential for success. A data-driven approach to customer feedback, where feedback is systematically gathered, categorized, and analyzed, provides deeper insights into user satisfaction and areas for improvement. Using automated tools to track surveys, NPS scores, or social media sentiment helps identify trends over time.
By integrating these insights into the product roadmap, product managers can align product features with customer desires, creating more user-centric experiences.
Eg: SaaS companies often use customer feedback to refine features, prioritize bug fixes, and guide the next set of product features.
3. Predictive Analytics for Market Trends
One of the most powerful tools in data-driven innovation is predictive analytics. By analyzing historical data, market conditions, and user behavior, product managers can forecast future trends and user needs. Predictive models provide the ability to anticipate market changes, optimize pricing strategies, and tailor marketing efforts accordingly.
For instance, Netflix uses predictive analytics to recommend shows based on viewing history, thus increasing user engagement and retention.
4. Data-Driven Personalization
Personalization is key to modern product experiences. Data-driven personalization involves tailoring products or services to individual user needs, behavior, or preferences. With machine learning algorithms and AI, product managers can create tailored experiences, enhancing user satisfaction and loyalty. A personalized approach to product offerings not only adds value to customers but also drives higher engagement and revenue.
Eg: Spotify utilizes data to personalize playlists, delivering tailored music recommendations to millions of users daily, enhancing the overall listening experience.
5. Data-Backed Hypothesis Testing (A/B Testing)
Data-backed hypothesis testing, such as A/B testing, is fundamental to data-driven innovation. In product management, A/B testing involves comparing two variations of a feature or interface to see which performs better with users. A/B tests provide statistically significant data to validate design changes, optimize user experiences, or refine features.
Product managers can experiment with different onboarding flows, pricing models, or UI changes to understand what works best for their target audience.
Eg: Google consistently uses A/B testing to improve user experience on its search engine, refining everything from search results to display ads.
6. Leveraging Big Data for Customer Insights
Big Data offers endless opportunities to uncover insights into customer behavior. By combining structured and unstructured data, companies can gain a comprehensive understanding of how users engage with products. Techniques such as sentiment analysis, social listening, and trend detection can be applied to large data sets to generate actionable insights.
By interpreting Big Data, product managers can identify customer pain points, anticipate needs, and adjust product strategy accordingly.
7. AI and Machine Learning for Automated Decision-Making
Artificial Intelligence (AI) and machine learning (ML) can help automate and enhance decision-making processes. These technologies analyze massive datasets quickly and provide insights that human analysis might miss. They can identify user patterns, detect anomalies, and even predict user behavior based on historical data, helping product managers stay proactive rather than reactive.
For instance, AI can be used to automate feature recommendations or even fine-tune pricing strategies in response to market dynamics.
8. Data-Driven Roadmapping
Data-driven roadmapping is about aligning the product roadmap with customer data, business goals, and market opportunities. It’s a strategic tool that ensures product decisions are grounded in data, not assumptions. Data helps prioritize which features will bring the most value, create product milestones, and guide the product’s evolution based on factual evidence.
With data-driven roadmaps, product managers are more likely to make decisions that are aligned with market demand, reducing risks and improving the chances of success.
9. Enhanced Product Performance Monitoring
Product managers can track how well a product is performing in real-time using data dashboards. Performance monitoring tools collect data on user engagement, conversion rates, churn, and other important metrics. This enables quick responses to underperforming features and ensures that products remain competitive.
The ability to monitor product health continuously is invaluable for making informed decisions on feature updates or when planning new product releases.
10. Cross-Functional Data Collaboration
For true data-driven innovation, product teams need to collaborate effectively with other departments, such as marketing, sales, and customer support, to share valuable insights and data points. Cross-functional collaboration ensures that everyone has access to the same data and that decisions are made based on a comprehensive understanding of the product’s ecosystem.
Effective collaboration reduces silos and allows for more holistic product innovation, making it easier to align strategy and execution across departments.
Conclusion
Data-driven innovation is more than just a buzzword; it’s a necessity in today’s fast-evolving digital landscape. By integrating real-time analytics, customer feedback loops, predictive analytics, and AI into product management, organizations can drive growth, optimize user experiences, and stay ahead of the competition. Product managers must continue to embrace data-driven methodologies to make informed decisions that not only meet current market demands but also anticipate future trends. The journey toward data-driven innovation is continuous, and those who stay on top of it will lead the way in the next wave of product development.
Embrace data-driven innovation today to shape a more informed and dynamic product future!
References
What is Data Driven Product Management?
https://productschool.com/blog/analytics/using-analytics-to-make-product-decisions